Welcome, Guest |
You have to register before you can post on our site.
|
Latest Threads |
What is the maximum numbe...
Forum: BDB Designer Q & A
Last Post: sariga.vr@bdb.ai
12-28-2022, 07:59 AM
» Replies: 0
» Views: 8,974
|
Inbuilt Capability of VC...
Forum: BDB - Platform
Last Post: shivani.jaipuria
12-27-2022, 05:23 AM
» Replies: 0
» Views: 1,594
|
Can dataset/cube refresh...
Forum: BDB - Platform
Last Post: shivani.jaipuria
12-27-2022, 05:08 AM
» Replies: 0
» Views: 1,625
|
How to load business stor...
Forum: BDB Designer Q & A
Last Post: sariga.vr@bdb.ai
12-26-2022, 04:47 PM
» Replies: 0
» Views: 3,820
|
How to load business stor...
Forum: BDB Designer Q & A
Last Post: sariga.vr@bdb.ai
12-26-2022, 04:46 PM
» Replies: 0
» Views: 3,942
|
How to load business stor...
Forum: BDB Designer Q & A
Last Post: sariga.vr@bdb.ai
12-26-2022, 04:45 PM
» Replies: 0
» Views: 2,919
|
How to load business stor...
Forum: BDB Designer Q & A
Last Post: sariga.vr@bdb.ai
12-26-2022, 04:44 PM
» Replies: 0
» Views: 2,917
|
Data Preparation operati...
Forum: BDB-Data Prep & ETL
Last Post: shivani.jaipuria
12-26-2022, 10:09 AM
» Replies: 0
» Views: 1,605
|
Plugability Feature of B...
Forum: BDB Platform Q & A
Last Post: shivani.jaipuria
12-26-2022, 08:32 AM
» Replies: 0
» Views: 1,470
|
How to use environment va...
Forum: BDB Platform Q & A
Last Post: archana
12-26-2022, 05:57 AM
» Replies: 0
» Views: 1,494
|
|
|
What is pipeline monitoring? |
Posted by: gnanashri - 12-23-2022, 05:40 AM - Forum: BDB - Data Pipeline
- No Replies
|
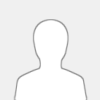 |
You may see details about the Pipeline components, Status, Types, Last Activated (Date and Time), Last Deactivated (Date and Time), Total Allocated and Consumed CPU%, Total allocated and Consumed Memory%, Number of Records, and Component logs in this page and can analyze and monitor them.
|
|
|
what is classification models and how do we measure the performance of models |
Posted by: manjunath - 12-23-2022, 05:34 AM - Forum: DS- Lab Q&A
- No Replies
|
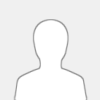 |
Classification models are a type of machine learning model that are used to predict a categorical output value based on one or more input features. They are commonly used in applications such as spam detection, credit fraud detection, and image classification.
There are many different types of classification models, including logistic regression, support vector machines (SVMs), and decision trees. The specific type of classification model that is most appropriate for a particular problem will depend on the characteristics of the data and the goals of the analysis.
To measure the performance of a classification model, there are several metrics that are commonly used. Some common classification evaluation metrics include:
· Accuracy: This is the percentage of correct predictions made by the model.
· Precision: This is the percentage of true positive predictions made by the model out of all positive predictions made by the model.
· Recall: This is the percentage of true positive predictions made by the model out of all actual positive cases.
· F1 score: This is the harmonic mean of precision and recall.
· AUC-ROC: This is the area under the receiver operating characteristic curve, which is a plot of true positive rate against false positive rate.
These metrics can be used to compare the performance of different classification models and to determine which model is the best fit for the data.
|
|
|
what are the different types of classification models |
Posted by: manjunath - 12-23-2022, 05:32 AM - Forum: DS- Lab Q&A
- No Replies
|
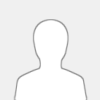 |
Classification models are a type of machine learning model that are used to predict a categorical output value based on one or more input features. There are many different types of classification models, including:
· Logistic regression: This is a linear model that is used to predict a binary outcome (such as 0 or 1). It is based on the logistic function and is used to model the probability of an event occurring.
· Support vector machines (SVMs): This is a linear model that is used to classify data points by finding the hyperplane that maximally separates the data points into different classes. It is particularly effective in cases where the data is not linearly separable.
· Decision trees: This is a non-linear model that is used to classify data points by recursively partitioning the data based on a set of decision rules. It is a simple and interpretable model that can handle both numerical and categorical data.
· Random forests: This is an ensemble model that is used to classify data points by combining the predictions of multiple decision trees. It is a powerful model that is resistant to overfitting and can handle high-dimensional data.
· Boosting: This is an ensemble model that is used to classify data points by combining the predictions of multiple weak learners. It is a powerful model that can handle complex data and can achieve high accuracy.
· Neural networks: This is a complex, non-linear model that is used to classify data points by learning a set of weights and biases through training. It is a powerful model that can handle complex data and can achieve high accuracy, but it can be resource-intensive and may require a large dataset for training.
|
|
|
Pareto Chart |
Posted by: aishwarya.rajan@bdb.ai - 12-23-2022, 05:32 AM - Forum: BDB Business Story Q & A
- No Replies
|
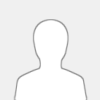 |
A Pareto chart is a type of chart that contains both bars and a line graph, where individual values are represented in descending order by bars, and the line represents the cumulative average.
Best Situation to Use a Pareto Chart is to identify the most frequent defects, complaints, or any other factor that the users can count and categorize to focus on where improvement efforts make the most impact.
There are two variations of the Pareto chart - Pareto Pyramid and Paired Pareto chart .
|
|
|
what is regression models and how do we measure the performance of model |
Posted by: manjunath - 12-23-2022, 05:29 AM - Forum: DS- Lab Q&A
- No Replies
|
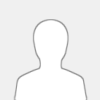 |
Regression models are a type of machine learning model that are used to predict a continuous output value based on one or more input features. They are commonly used in applications such as prediction, forecasting, and trend analysis.
There are many different types of regression models, including linear regression, logistic regression, and support vector regression. The specific type of regression model that is most appropriate for a particular problem will depend on the characteristics of the data and the goals of the analysis.
To measure the performance of a regression model, there are several metrics that are commonly used. Some common regression evaluation metrics include:
· Mean Absolute Error (MAE): This is the average absolute difference between the predicted values and the actual values.
· Mean Squared Error (MSE): This is the average squared difference between the predicted values and the actual values.
· Root Mean Squared Error (RMSE): This is the square root of the MSE.
· R-squared: This is a measure of how well the model fits the data, with a value of 1 indicating a perfect fit and a value of 0 indicating no fit.
* Adjusted R-squared: This is a modified version of R-squared that takes into account the number of variables in the model
|
|
|
what are different types of regression models available . |
Posted by: manjunath - 12-23-2022, 05:23 AM - Forum: DS- Lab Q&A
- No Replies
|
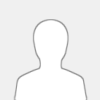 |
Regression models are a type of machine learning model that are used to predict a continuous output value based on one or more input features. There are many different types of regression models, each with its own strengths and weaknesses. Here are some common types of regression models:
1) Linear regression: This is a simple regression model that assumes a linear relationship between the input features and the output. It is used to predict a continuous output value based on a single input feature or a linear combination of input features.
2) Logistic regression: This is a regression model that is used to predict a binary outcome (such as yes/no or 0/1) based on one or more input features. It is used in classification tasks and is based on the logistic function, which maps the input features to a probability between 0 and 1.
3) Polynomial regression: This is a regression model that is used to fit a non-linear relationship between the input features and the output. It is based on a polynomial function and can be used to fit higher-order relationships in the data.
4) Ridge regression: This is a regularized version of linear regression that is used to prevent overfitting. It adds a penalty term to the loss function to constrain the size of the coefficients and reduce the complexity of the model.
5) Lasso regression: This is another regularized version of linear regression that is used to prevent overfitting. It adds a penalty term to the loss function to constrain the size of the coefficients and reduce the complexity of the model.
6) Elastic Net: This is a combination of ridge and lasso regression that combines the penalties of both models. It is used to balance the trade-off between the simplicity of the model and the fit of the model to the data
|
|
|
|